Selected Projects
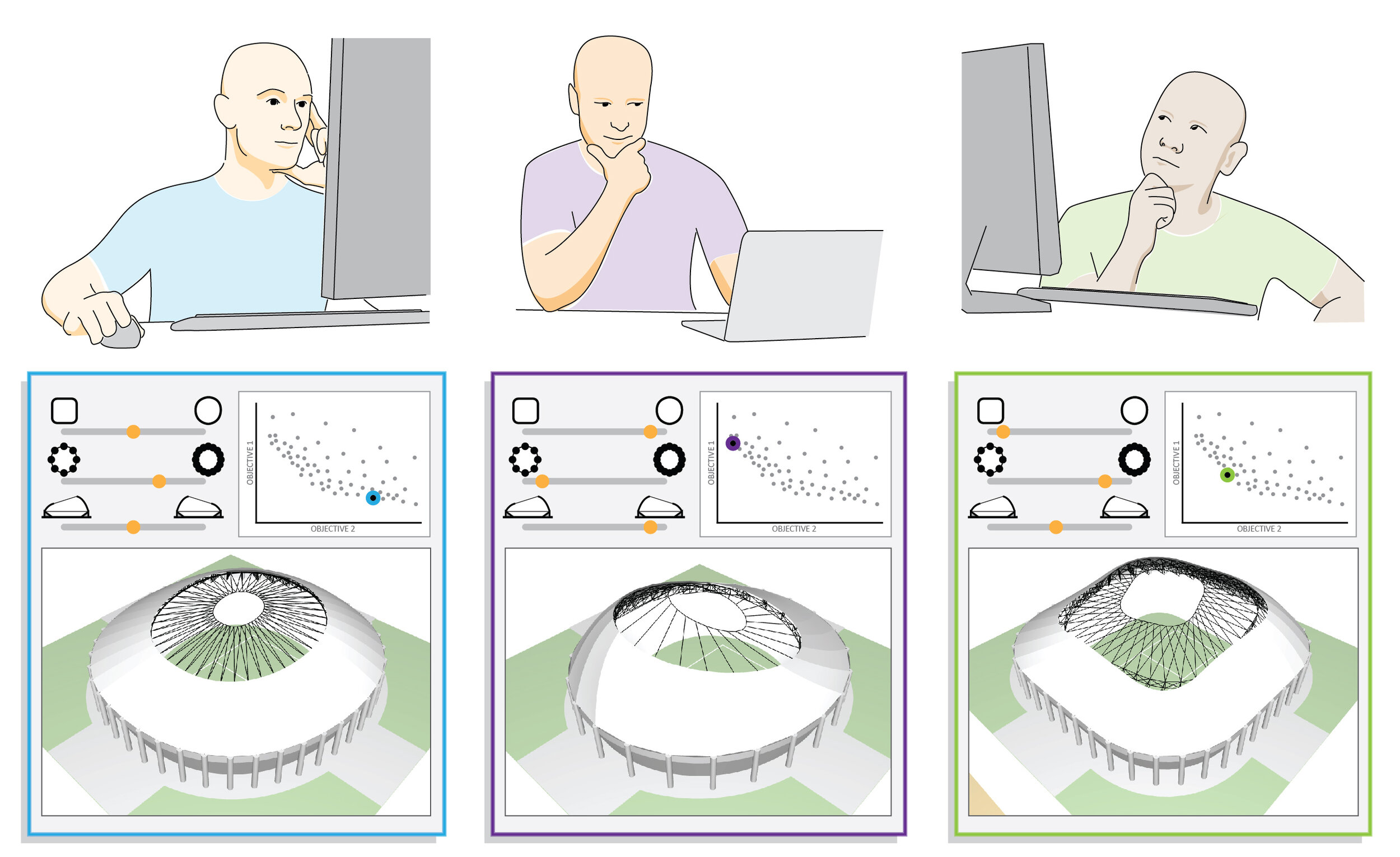
Characterizing Expert Behavior During Interactive Parametric Building Design
Optimization techniques within parametric models can systematically find the best options for quantitative design goals such as energy use or structural efficiency. However, there is considerable uncertainty about how to best apply optimization, especially for emerging interactive design approaches that let designers manage qualitative and quantitative goals simultaneously. While existing literature describes designer behavior in general, little is known about how these emerging human-computer collaborative tools influence design, how cognitive processes relate to the outcomes they produce, and especially how they are enacted in the domain of architectural engineering. The goal of this NSF-funded project is to characterize the behavior of expert designers in the AEC industry while they employ early, optimization-based parametric design methods to formulate and explore design options.
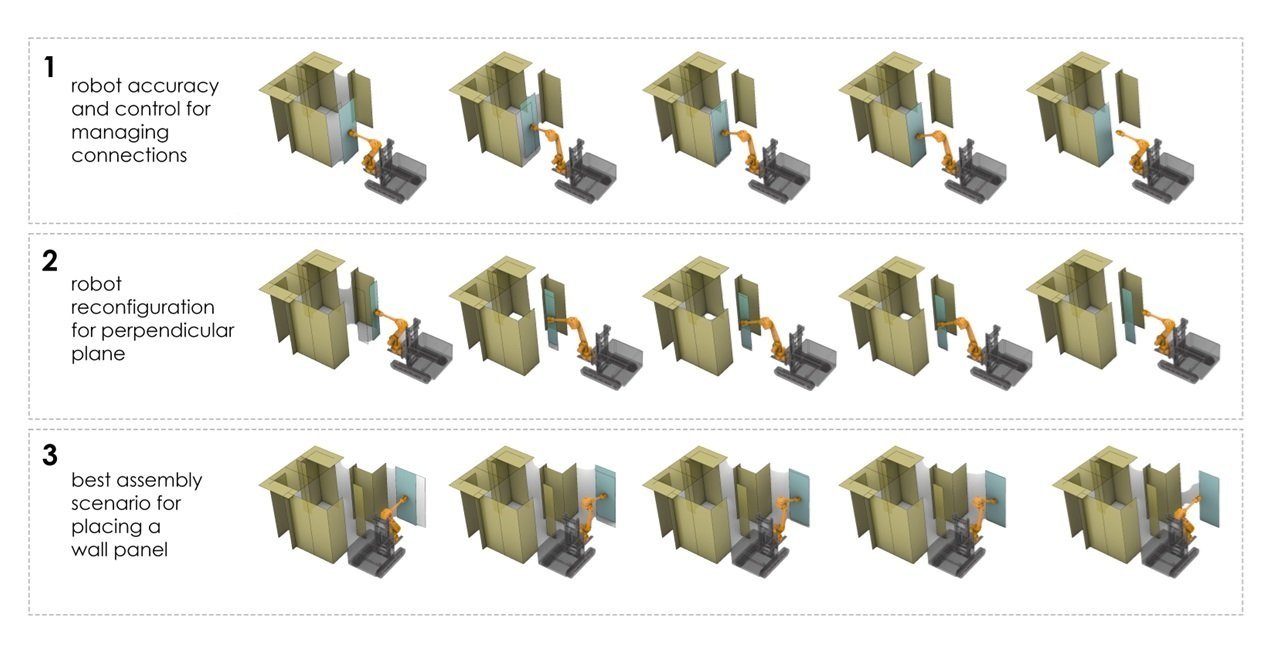
Leveraging Robotic Constructability Assessment in Early Design
Early simulations predicting building behavior in terms of structure, energy, and daylight can help designers prioritize performance goals--yet it is difficult to quantify how easy something is to build at this stage. The advent of robotic construction provides an opportunity to experiment with constructability assessment in early computational design settings, since parametric simulations of possible robot movements create datasets that can be mined for insights into the construction process. This project is defining and testing quantitative assessments of constructability while demonstrating how new constructability metrics can shift optimal design outcomes.
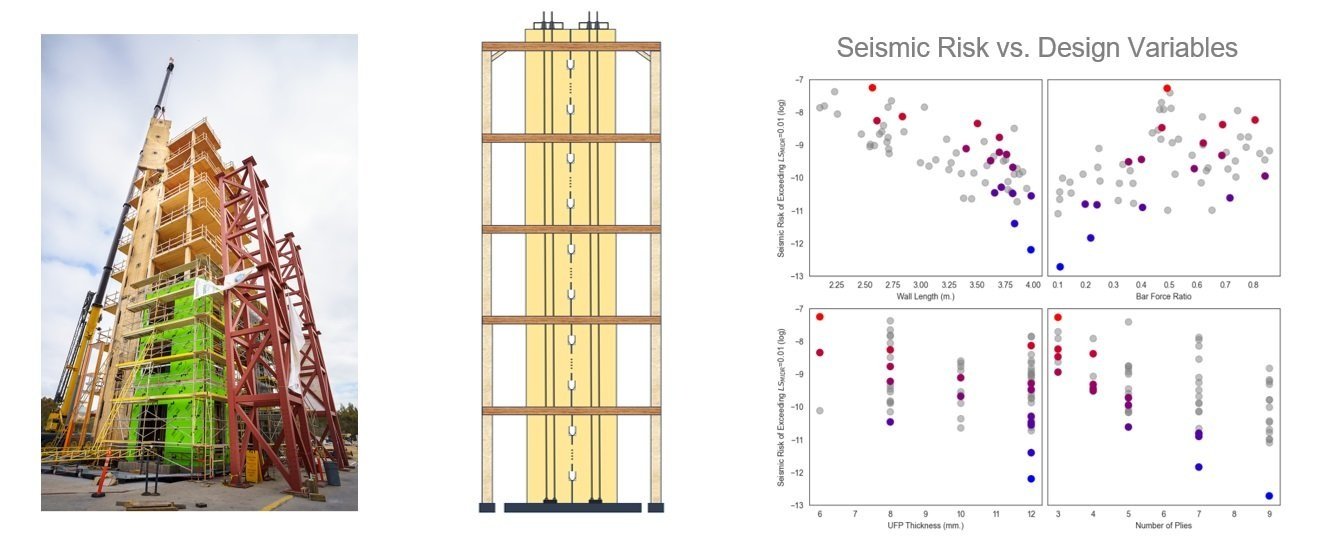
Converging Seismic Design Optimization
The design of lateral systems for mass timber buildings in earthquake-prone areas involves careful consideration of both seismic performance and sustainability. Due to the extensive uncertainty and corresponding computational requirements of simulating earthquakes, it can be difficult to determine how design variables might affect both goals. In this project, we are developing optimization procedures designing mass timber lateral systems based on consensus-based functional recovery and sustainability metrics. This NSF-funded research is a collaboration with the NHERI Converging Design Team, which includes researchers from Oregon State, Colorado State, and Stanford.
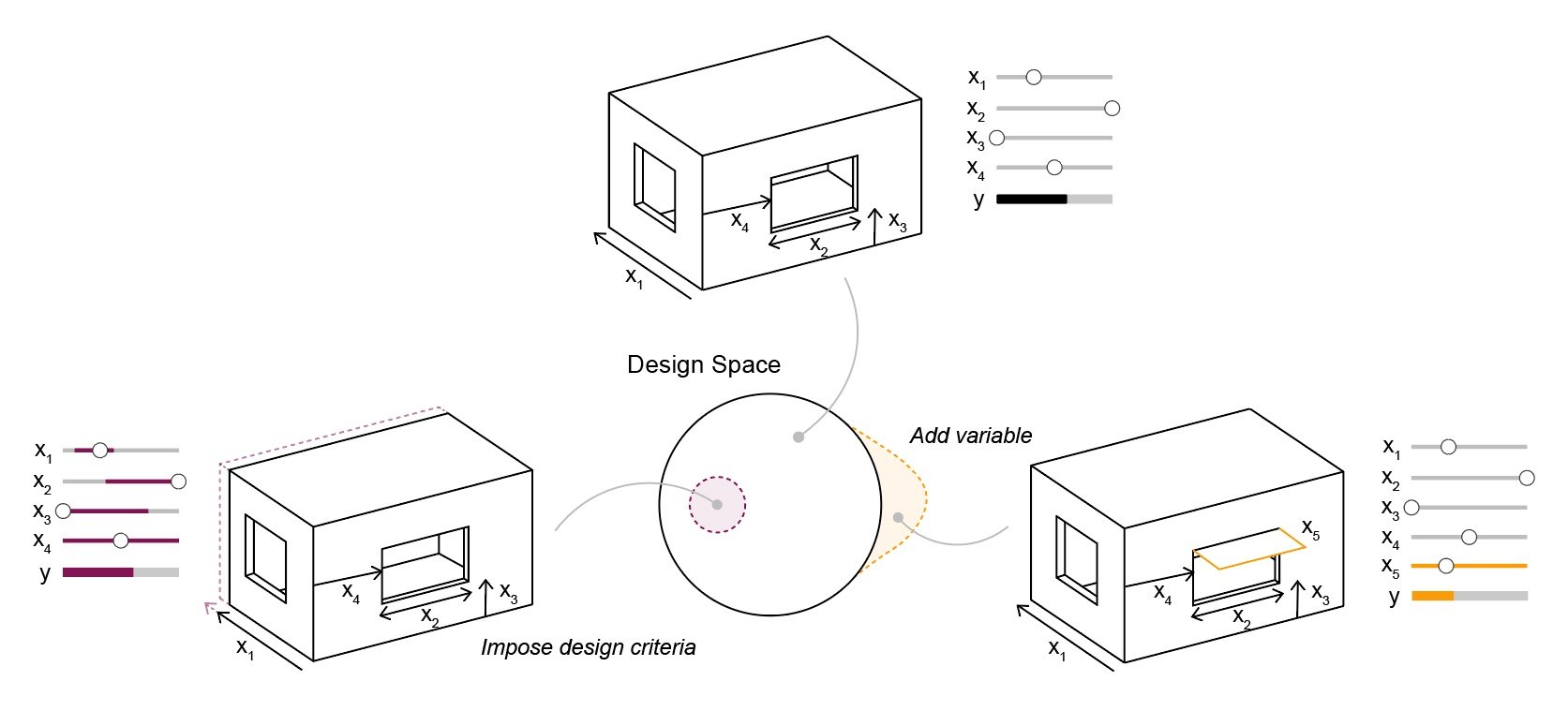
Flexible, Timeline-Responsive Parametric Design
Many effective design space exploration methods have been proposed for building design problems. However, many of these methods have not yet been widely adopted, despite their potential to positively influence performance, particularly in the early stages when the design is most adjustable. This research considers that existing performance-based parametric approaches often require too much customized effort to be accessible and are not flexible enough to provide feedback and guidance at crucial points when designers really need them. This research proposes developing more generalizable design spaces that can be adjusted during the design process by imposing design criteria or adding new variables. Through applying statistical/ML methods, insights can be extracted that are directly conscious of the design timeline.
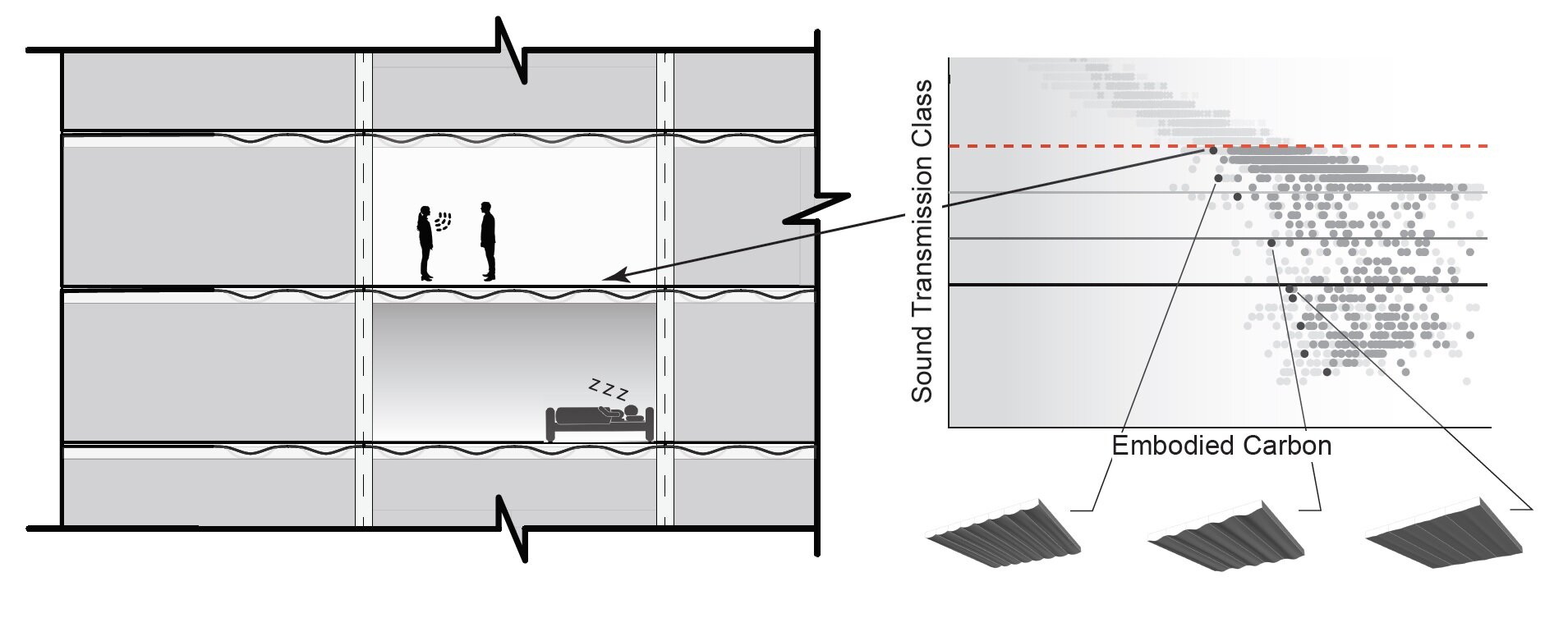
Structural + Acoustic Optimization of Shaped Floors
With advancements in digital design tools, architects and engineers have developed novel sustainable building components that reduce the amount of embodied carbon in structures while maintaining structural integrity. However, these optimized structures can neglect secondary design objectives such as acoustics during the computational process. This research investigates the relationship between structural and acoustic performance for shaped concrete slabs with curved, non-standard geometry. Design space exploration techniques such as sampling, multi-objective optimization, and constrained optimization are used to find broad structural-acoustic trends, identify a Pareto front, and identify the best performing shaped slabs at varying acoustic performance levels.
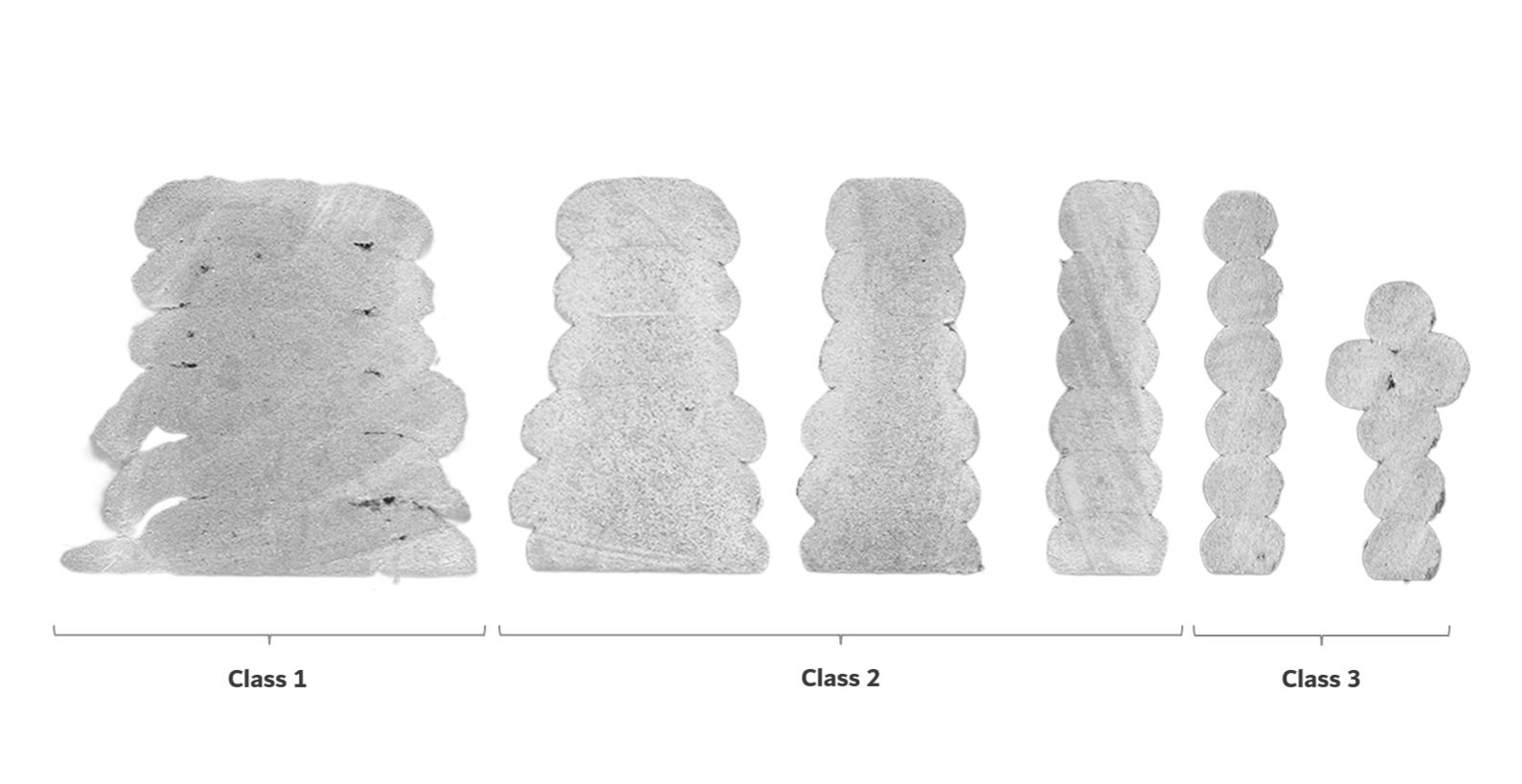
Predicting the Quality of 3D Concrete Prints
3D concrete printing (3DCP) has emerged as an innovative technology that can offer numerous benefits over traditional building methods, including the ability to produce unique structures with high accuracy and precision, reduce construction time and costs, and minimize environmental impact. However, for 3DCP to become a reliable construction technique, additional research and development are necessary in both material design and printing systems. This project seeks to improve the reliability of the 3DCP system through filament shape simulation, which helps in optimizing the printing parameters, printing filaments with varying width, and printing non-planar layers. The goal is to develop a fast and accurate data-driven model that can predict filament shape based on robot speed, material flow rate, nozzle height, and diameter. While this model has the potential to be applied to any 3DCP project, it can only be used with buildable materials.
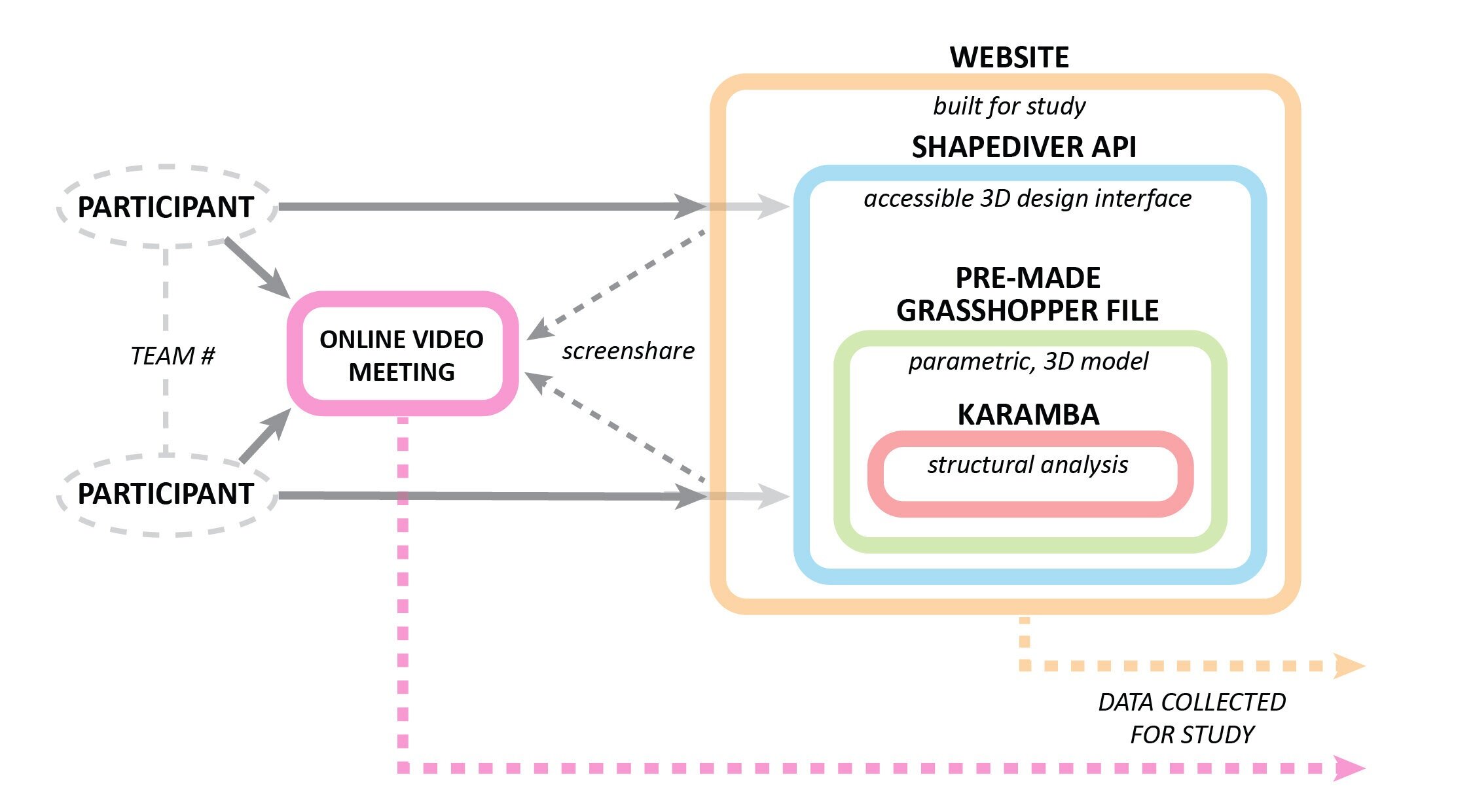
Digital Collaboration between Architects and Engineers
Architects and engineers often have to work together to design and construct buildings, but differences in their education, vocabulary, tools, and goals can often make collaboration difficult. As design increasingly occurs largely or entirely within digital environments, computational design tools can either help or hurt, as diversity in teams may foster better performance as long as the environment is conducive to shared understanding. In a series of studies, we consider interactions between teams of architects and engineers while working together towards a conceptual design while assessing confidence, efficacy, quality of interaction, and other aspects of the design process.
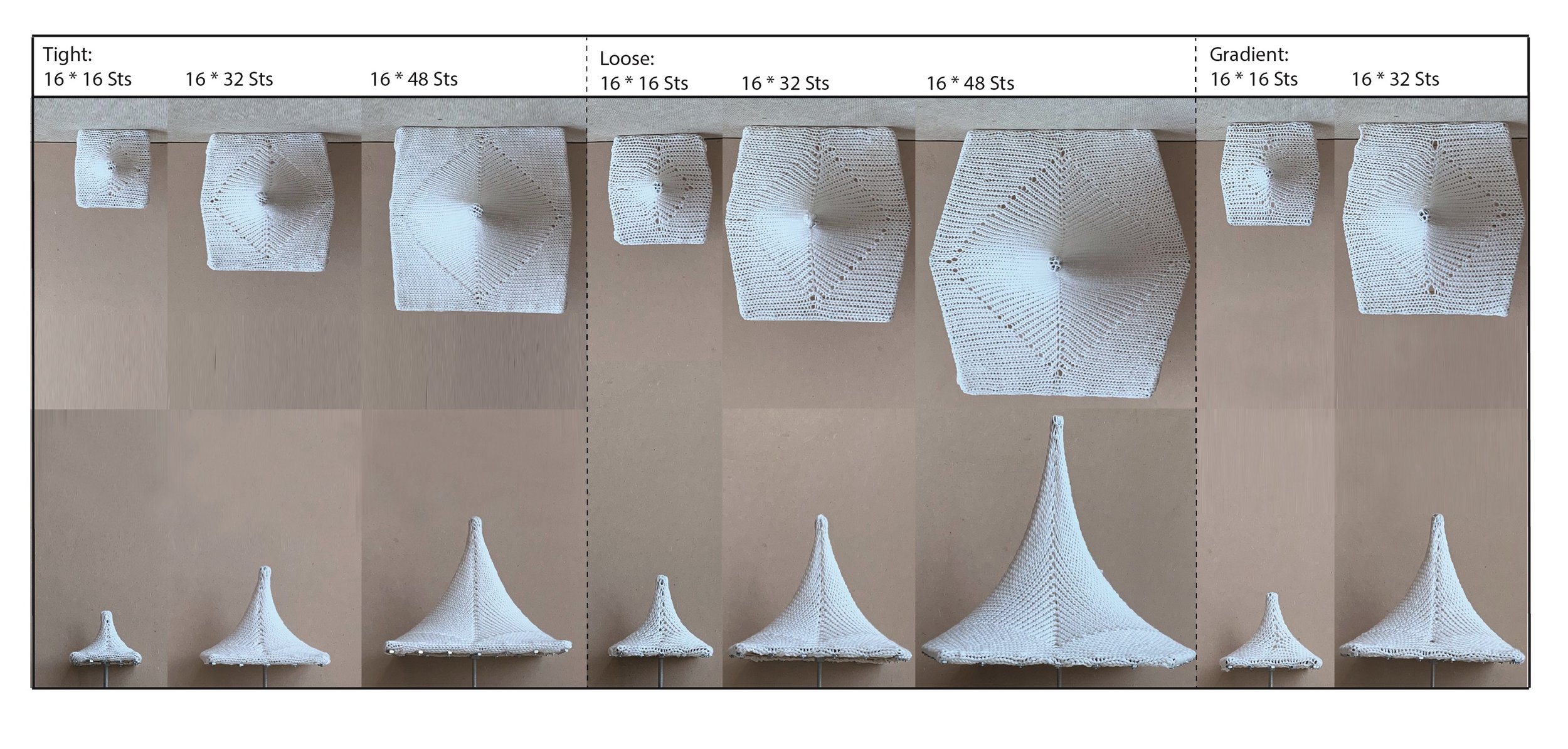
ML-based Analysis and Reverse Form-Finding of Architectural Knitted Textiles
Knitted materials are not commonly used in the design of architectural tension structures, and while they offer benefits, their successful integration at the architectural scale requires critical analysis of the material's behavior. However, requirements for manual manipulation of computational form-finding tools and sophisticated structural analysis can be limiting to design exploration. The goal of this project is to develop machine learning (ML) models, often from experimentally generated data, that can reduce tedious tasks in designing with knit. Various ML approaches help to map between the inputs and outputs of different steps of the design process, such as setting the form-finding parameters for a computational model of a given knit pattern, and translating a final form into the initial shape and settings required for manufacturing.
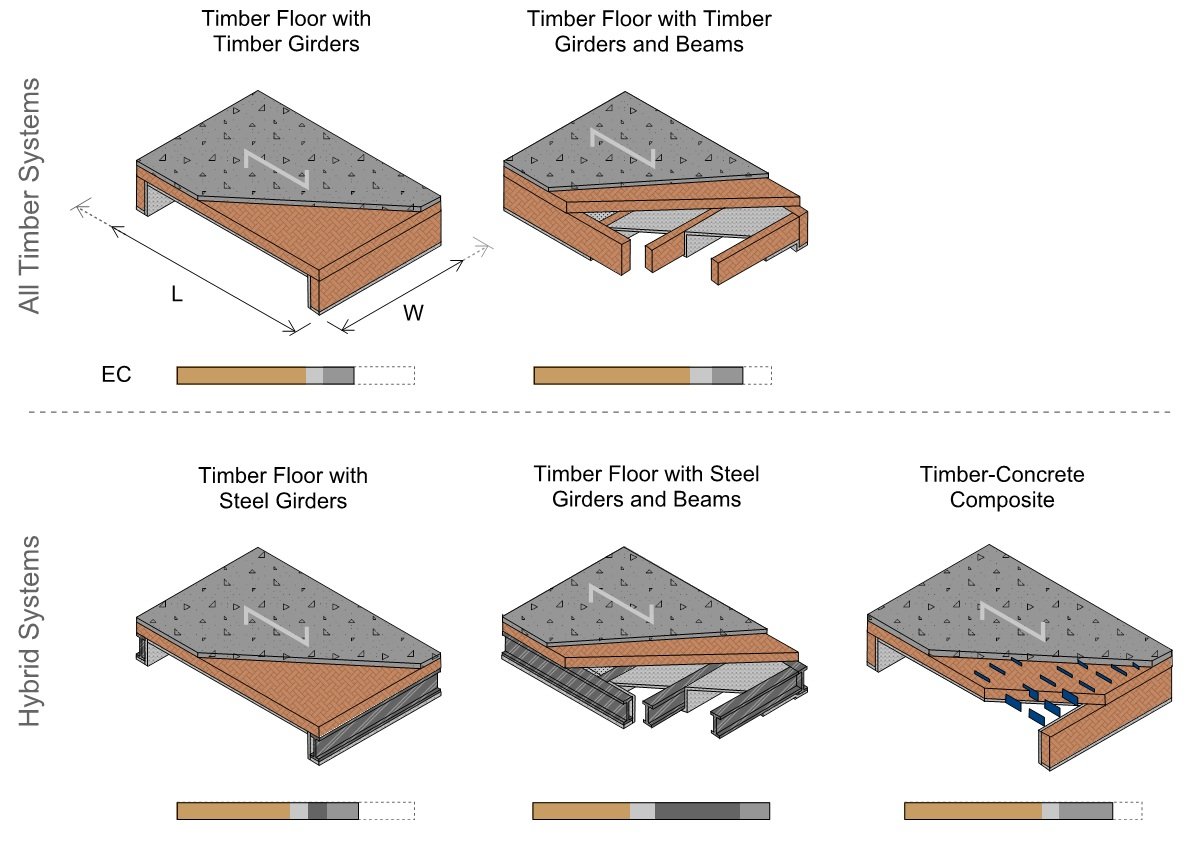
Embodied Carbon of Timber Floors
Structural mass timber has been used more often in buildings over the last five years given its potential to reduce embodied carbon (EC). Decisions made during a project's early design stage can affect the final embodied carbon of the structure; however, there is limited guidance for designers to help guide those early decisions, specifically related to selecting floor system types, fire protection strategies, and bay layouts. A parametric analysis was performed in this project to explore the effects of these parameters on embodied carbon for a variety of all-timber and hybrid-timber floor systems. This project aims to produce an underlying data set that could be used to develop design aids and tools for early design.
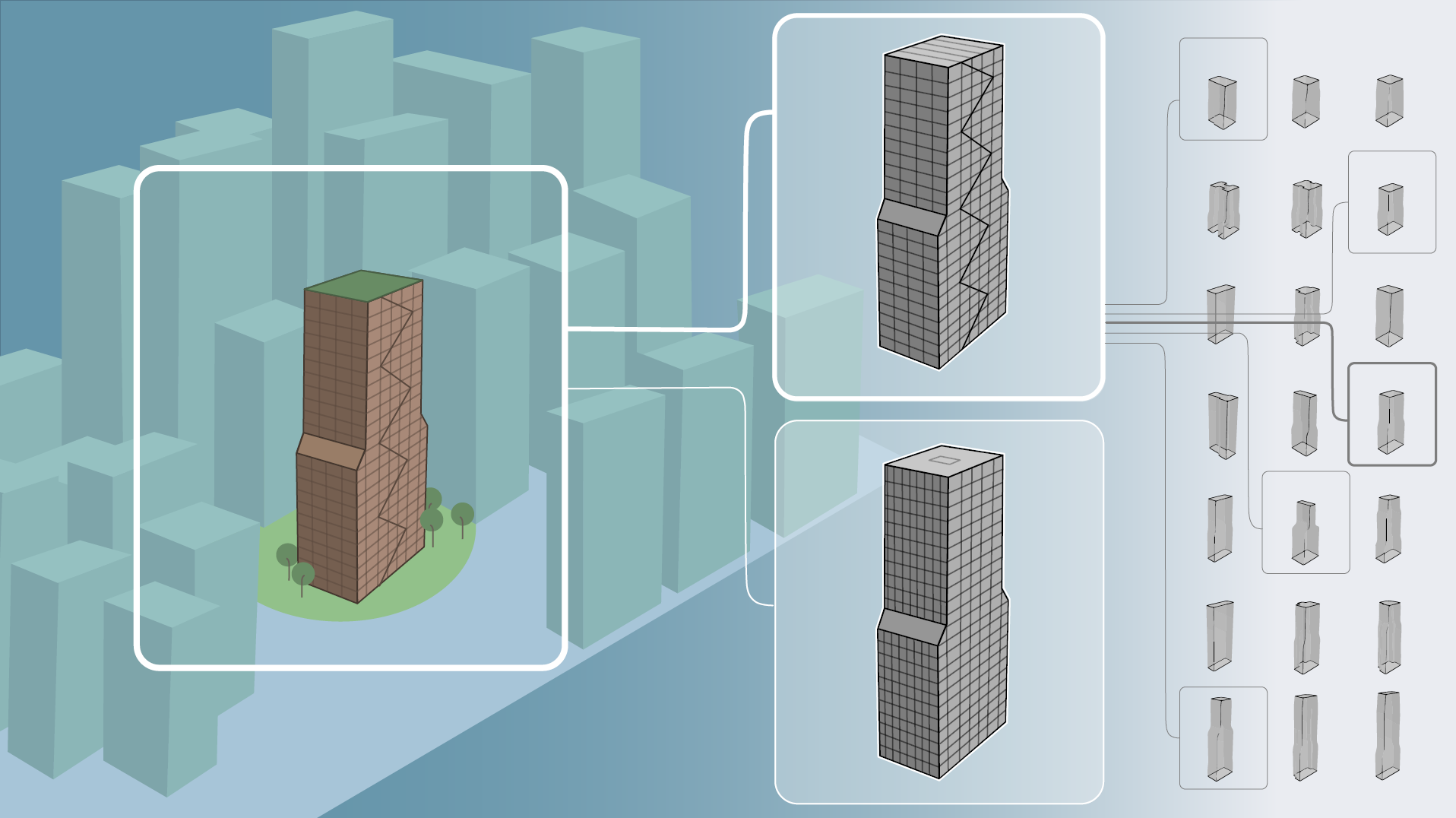
Design Space Exploration for Tall Timber
As demonstrated by several notable precedents around the world, timber structural systems are becoming viable for taller and taller buildings. However, we are still learning about the design possibilities of wood at this scale. This project uses design space exploration to consider the relationship between geometric design decisions and performance for tall timber buildings. A parametric framework was developed that performs automated structural design while considering typical loads, material properties, and architectural requirements. This framework was used to explore diverse timber design spaces including separate systems, footprints, notches, and tapers. Trends in feasibility and embodied energy are analyzed across this range of geometries. By providing feedback for early-stage design, this research aims to increase the application of timber as a structural material for large-scale construction.
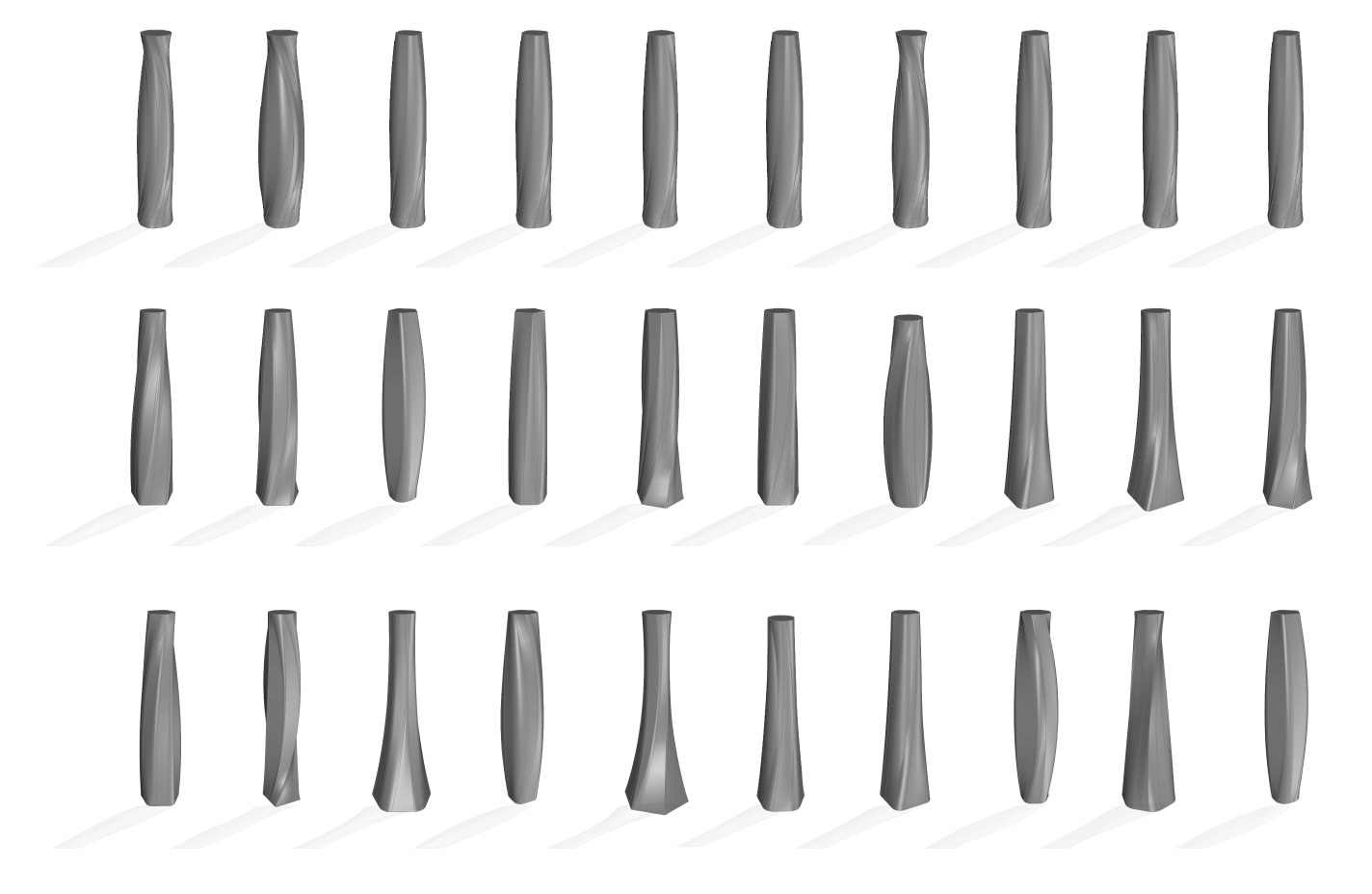
Developing Approaches to Diversity-Driven Parametric Design
To be useful for architects and related designers searching for creative, expressive forms, performance-based digital tools must generate a diverse range of design solutions. This gives the designer flexibility to choose from a number of high-performing designs based on aesthetic preferences or other priorities. However, there is no single established method for measuring, diversity in the context of computational design, especially in the field of architecture. This project explores different metrics for quantifying diversity in parametric design, which is an increasingly common digital approach to early-stage exploration, and tests how human users perceive these diversity measurements.
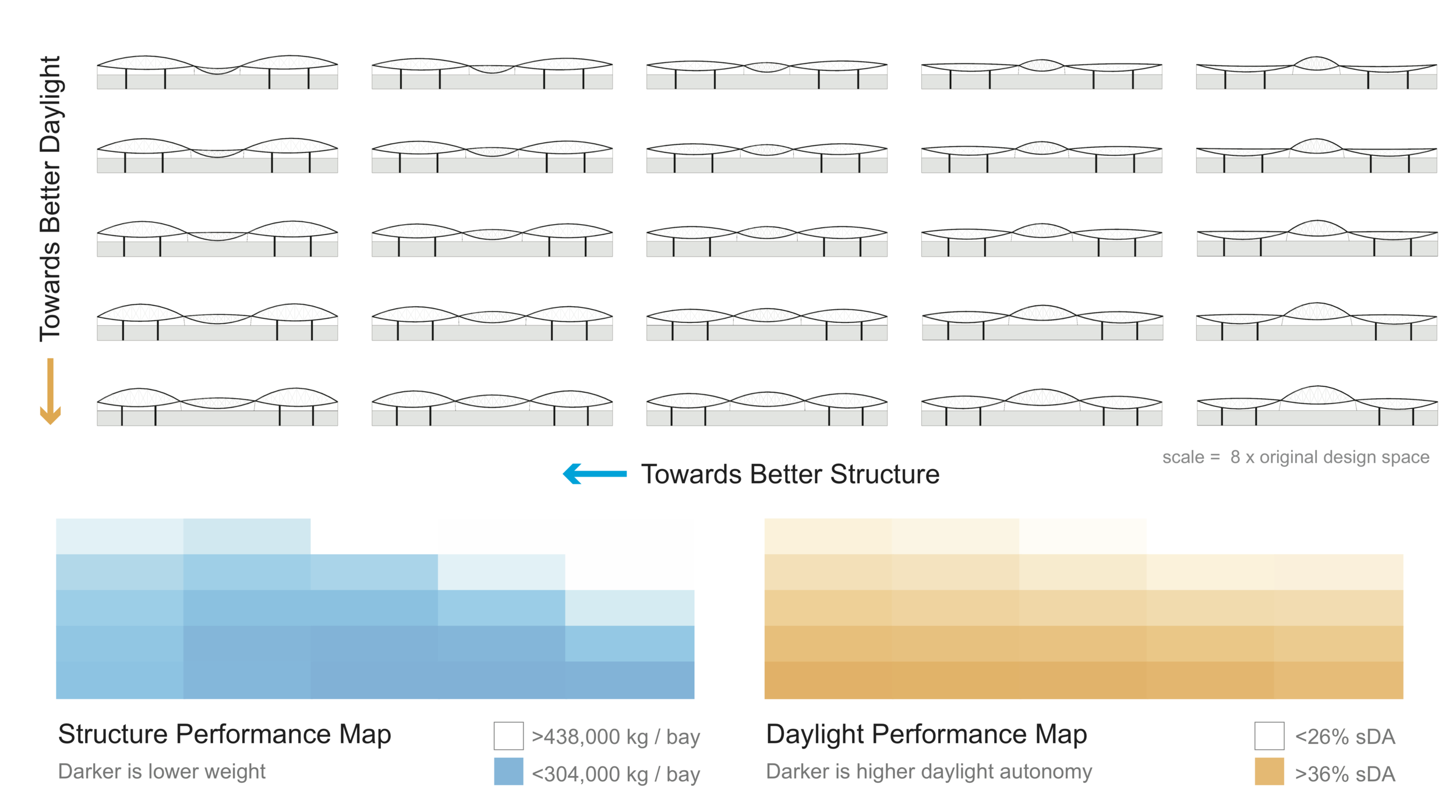
Using ML to Find Useful Design Space Patterns
Many architectural designers recognize the potential of parametric models as a worthwhile approach to performance-driven design. A variety of performance simulations are now possible within computational design environments, and the framework of design space exploration allows users to generate and navigate various possibilities while considering both qualitative and quantitative feedback. At the same time, it can be difficult to formulate a parametric design space in a way that leads to compelling solutions and does not limit flexibility. This project proposes and tests the extension of machine learning and data analysis techniques to early problem setup in order to interrogate, modify, relate, transform, and automatically generate design variables for architectural investigations.
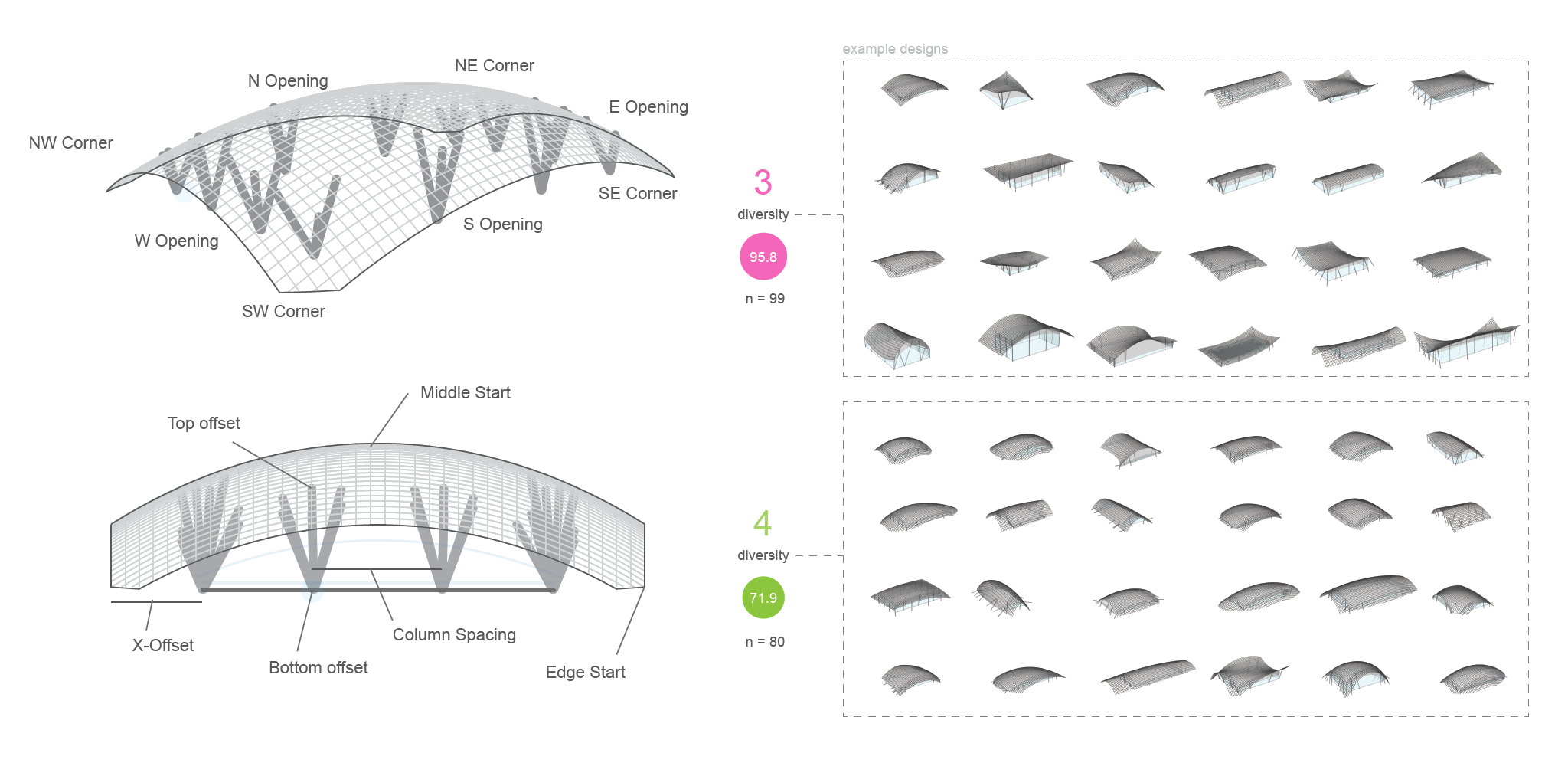
Studying the Effect of Computational Tools on the Design Process
Various interactive optimization techniques have been proposed to simultaneously consider quantitative and qualitative goals in conceptual building design. However, more research is required to understand the effects of these techniques on design processes and outcomes at the building scale, when more substantial simulations are considered. This area of research focuses on how simulation-based, data-driven design tools influence design processes in terms of performance, designer preferences, and creativity.